Fantastic Web 3 Advocates and Where to Find Them - Part 1 of 2
Table of Contents
Part 1
Part 2
Important Disclaimer
1. Introduction
A project kicked off and devs are building. The founders want people to know about their project, so they decide to tap L1 partners, VC investors, and retail advocates to help them showcase the projects—but out of thousands of advocates, whose doors should they knock on?
Founders are advised to partner with a Layer 1 Foundation that is highly supportive in building a community, yet there is no conclusive proof for them to verify the claim-to-be-supportives. Moreover, being supportive could very much digress from being actually helpful; its assistance could well be fruitless. All these questions are often left unanswered, or are answered in subjective forms.
We will try to provide a rule-of-thumb for choosing marketing partners, solely regarding the community power. What we WON’T provide: “A chain is superior to B chain, so choose A.” Instead, what we will provide: “A chain has been more supportive and more effective in a project’s Twitter growth than B chain, so choosing A is advisable if your priority resides in the Twitter community.”
In our previous paper, ‘The Hitchhiker’s Guide to Web3 Marketing’, we specifically listed out ready-to-use marketing tactics to be administered on Twitter, and emphasized on how it is important to message ‘legitimacy.’ Along the course, we also suggested making use of L1 and VC partners—or, third party agents— to convey the message.
As a follow-up for the guidance, we quantified the effect of advocacy shoutouts by various categories, and with this data, will try to provide a criteria for L1s, VCs, and retail advocates regarding choosing the right partners for community building.
In short, this paper will provide a guideline on which marketing partners should founders go for, when the founders are looking to improve upon their Twitter presence.
2. Data Specification
2.1. Data Collection
All our datasets in this paper were collected using the Twitter API. The scraped data comprises the following:
- – Name (ex. Factomind)
- – Handle (ex. @factomind)
- – Location
- – Description
- – Follower
- – Following
- – Listed
- – Posting
- – Account Creation Date
- – Verified
- – Like
- – Retweet (Reply)
We collected above data points for ~4,000 advocate handles and ~250 project handles. For advocate handles, we surveyed 600+ Twitter crypto lists and gathered respective list memberships.
From the above data points, we derived the following community metrics called interactions. For a detailed definition of interactions, please refer to our previous paper here.
From the above metrics, we derived derivative metrics as below:
- D0 = The day before a Tweet
- Influential FactorD1 = The rate of growth in interaction on the day of a Tweet
- Influential FactorD2 = The rate of growth in interaction after a day from a Tweet
- Interaction ChangeD1 = The growth in interaction on the day of a Tweet
- Interaction ChangeD2 = The growth in interaction after a day from a Tweet
Our final advocate dataset would look like below.
For our data for Social Media volumes, we used data obtained from major data vendors and cross-checked with our internal database. No raw data has been used since we used derivative statistics instead. An example time series for our Social Media Volume data would look like below:
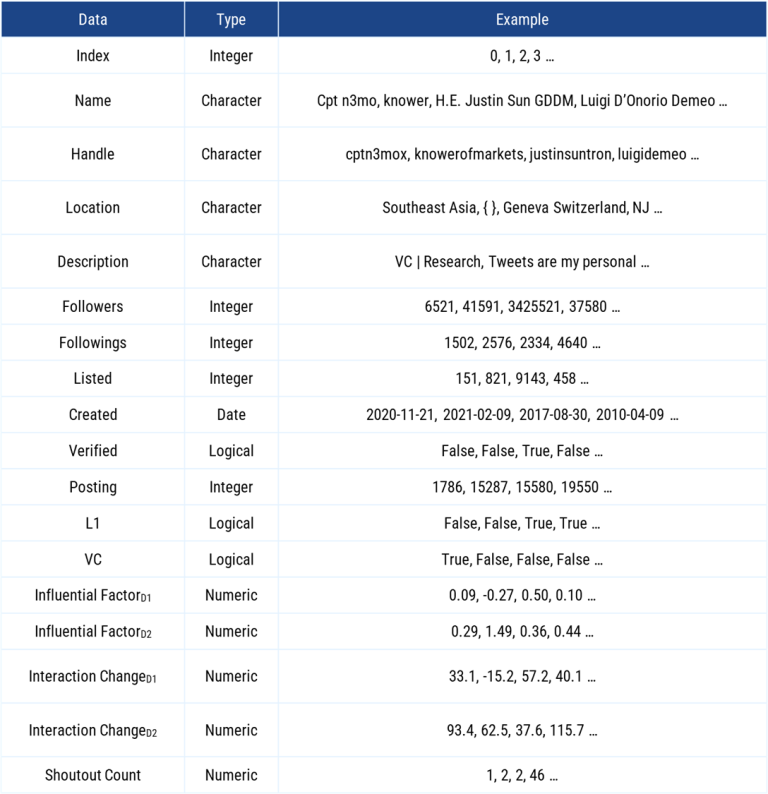
Table 1 – The Dataset Description of 3,802 Advocates
2.2. Research Methodology
Consideration 1 : All interactions to be counted
We used interactions, not organic interactions, for computing our derivative metrics (influential factor, interaction change) because in this paper, our focus is to quantify the impact of the shoutouts from advocates, rather than measuring the soundness of a project’s community itself.
Consideration 2 : Direct mentions only
Consideration 3 : Echos only
Plus, the project itself has to Reply to or RT the exact shoutout Tweet, or the Tweet thread header containing the shoutout, for the shoutout to be counted in our analysis. This process was taken only to simplify data collection.
Consideration 4 : Identification of Twitter handles
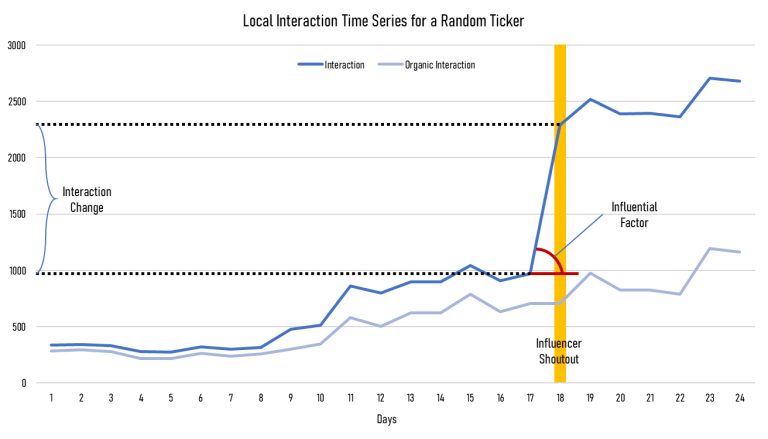
Figure 1 – How to Calculate Interaction ChangeD1 and Influential FactorD1